Algorithmic Marketing Training Boot Camp
- Course Duration3 Months
- Course StartEnrollment Monthly
Description
What is Algorithmic Marketing?
We define algorithmic marketing as a marketing process that is automated to such a degree that it can be steered by setting a business objective in a marketing software system. This implies that the marketing system should be intelligent and knowledgeable enough to understand a high-level objective, such as the acquisition of new customers or revenue maximization, to plan and execute a sequence of business actions, such as an advertisement campaign or price adjustment, with the aim of achieving the objective, and to learn from the results to correct and optimize the actions if needed. In this course, we also use the term programmatic to refer to highly automated marketing software systems and services, and the terms algorithmic and programmatic are used interchangeably in most contexts.
Who should attend?
Adverting Agency Executives, Media Planners, Media Buyers, Implementers of marketing software, Marketing Managers, Product managers, Finance managers and software engineers who want to learn about the features and techniques that can be used in marketing software products and also learn about the economic foundations for these techniques.
The other target audience are Market Research Executives, Media Research, Marketing strategists and Technology leaders who are looking for guidance on how marketing organizations and marketing services can benefit from machine learning and Big Data and how modern enterprises can leverage advanced decision automation methods.
Tools to be used
- Python
- GIS
Machine Learning and Predictive Modelling Introduction
This training looks at basic methods of machine learning and economic modelling that enable predictive analysis and its building blocks. Focus will be to describe the main capabilities and limitations of predictive modelling, rather than to provide a comprehensive study of machine learning algorithms.
Promotions and Advertisements
This training focuses on targeting as a problem of customer experience optimization that is driven by a mix of multiple business objectives and controls many different marketing activities. It begins with an overview of the retail promotion environment that will help you to better understand the problem of targeting. It then describes a promotion targeting framework that includes a more formal definition of business objectives, basic building blocks of behavioural modelling, and more complex constructs used in marketing campaigns. After that, it looks at online advertising environment and related targeting methods. Although the retail and online advertising environments complement each other and many targeting methods are universally applicable, we will study them separately because of major structural differences and variations in objectives. Measurement plays an extremely important role in all marketing applications, and the framework that we develop will also be used in other programmatic services, including search, recommendations, and pricing.
Search
In this training, we will look at first product discovery service which is search. The purpose of search services is to fetch offerings that are relevant to the customer’s search intent expressed in a search query or selected filters. Search services solve the problem of product discovery, which can be viewed as a particular case of targeting. We will be taking a practical approach to search methods and will focus on industrial experience, techniques, and examples, rather than information theory. At the same time, we will try to avoid implementation details, such as data indexing, as much as possible and will stay focused on the business value delivered through relevant search results. We will start this training with a review of the environment and economic objectives. We will then demonstrate that the problem of relevant search can be expressed in terms of features, signals, and controls, similarly to other programmatic services. After that, we will review a number of methods for engineering, mixing, and tuning these signals and controls in manual mode, and we will then discuss how predictive analytics can be leveraged for automated optimization.
Building Recommendations engines
Digital channels enable marketers to carry extremely wide and deep assortments with a large number of slow-moving niche products. This is one of the key differentiators in a comparison with traditional distribution channels, where the assortment is limited by distribution costs. Extremely wide and deep assortments with a long tail of niche products create a need for efficient discovery services, including search and recommendation. Recommendation services, in contrast to search services, aim to provide the customer with relevant offerings when a search intent is not or cannot be clearly expressed. Also, recommendation systems can generally leverage user–item interaction data, which include explicitly provided ratings and implicitly collected browsing histories, catalogue data, and contextual information. The main output of the recommendation service is a ranked list of recommended items Therefore, this training systematically describes recommender systems by starting with the environment and economic goals and then diving deeper into various recommendation methods.
Pricing and Assortment
The last part of this training we will look at the problem of price management. We will start with a review of the basic principles of strategic pricing and price optimization. We will then continue with the development of more tactical and practical demand prediction and price optimization methods for market segmentation, markdowns, and clearance sales. We will also briefly review the major resource allocation methods used in service industries to set booking limits. Finally, we will consider the assortment optimization problem, for which we can reuse some of the building blocks developed for price management.
Our Partners
Institutions we have partnered with or Worked with previously
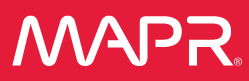
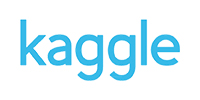
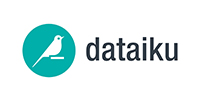
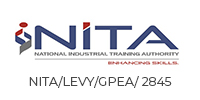
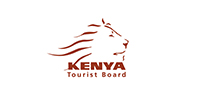
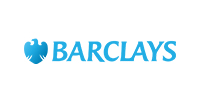
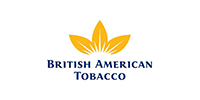
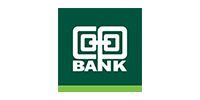
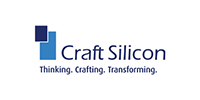
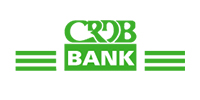
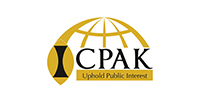
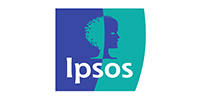
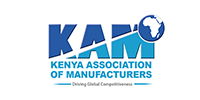
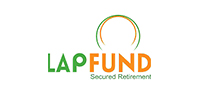
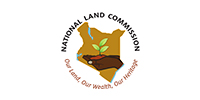
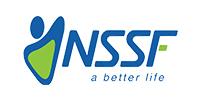
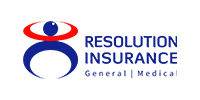
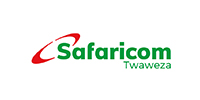
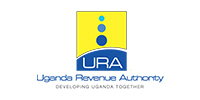